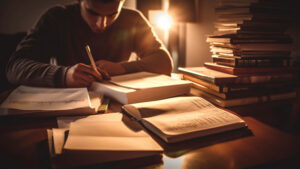
Table of Contents
Artificial intelligence (AI) is revolutionizing the field of radiation oncology, transforming the way radiation oncologists treat cancer patients. By leveraging recent advances in AI algorithms and machine learning techniques, AI enhances treatment planning and delivery in radiation oncology, ultimately improving patient outcomes. With its ability to optimize treatment accuracy, efficiency, and personalized care, AI in radiation oncology holds immense potential for medical physicists.
The integration of artificial intelligence (AI) technology allows for adaptive radiation therapy, enabling precise dose distribution tailored to individual patients. This breakthrough has significant implications for various types of radiotherapy treatments such as prostate and neck cancer radiotherapy. By reducing human operator error and enhancing decision-making processes, AI implementation in radiation oncology empowers medical physicists and radiation oncologists to leverage recent advances in radiomic features and imaging.
Through the use of magnetic resonance imaging and other cutting-edge technologies, artificial intelligence (AI) assists radiation oncologists in creating more effective treatment plans for adaptive radiation therapy. This state-of-the-art approach has the capacity to transform how radiation oncology approaches cancer treatment, minimizing side effects.
State of the Art: Recent Advances in AI for Radiotherapy
Recent advancements in artificial intelligence (AI) have revolutionized the field of radiation oncology, bringing about significant improvements in tumor detection and segmentation accuracy. By harnessing machine learning algorithms, radiation oncologists can now analyze vast datasets comprising CT, MR images, and more to identify patterns and predict treatment responses with remarkable precision.
Deep learning models, powered by artificial intelligence (AI), have emerged as a particularly promising approach in automating various aspects of radiation oncology. These models leverage neural networks (DNN) to learn from large amounts of data, enabling them to perform tasks such as organ-at-risk segmentation or treatment plan optimization[^3^].
Moreover, advanced image registration techniques have facilitated the precise alignment of multiple imaging modalities, including ct images. This capability is invaluable when integrating information from different scans or time points, allowing clinicians to create a comprehensive understanding of the patient’s condition. These applications of image registration contribute to the optimization of adaptive radiation therapy.
Applications of AI in Image-Guided Radiotherapy
Artificial intelligence (AI) revolutionizes the field of radiation oncology by enhancing accuracy, efficiency, and adaptability in treatment planning and delivery. AI is making significant strides in Image-Guided Radiotherapy (IGRT), particularly in the analysis of CT images. Let’s explore how AI is transforming the imaging aspect of IGRT.
-
Artificial intelligence (AI) has revolutionized patient care with its deep learning capabilities. Automated image analysis, one of the many applications of AI, utilizes AI-powered algorithms to analyze medical imaging, specifically CT images. This advanced technology enables accurate target delineation during treatment planning, streamlining the process and minimizing the potential for human error.
-
Artificial intelligence in radiotherapy is advanced and has promising future prospects. One of its remarkable features is real-time tracking. By utilizing machine learning algorithms and the power of artificial intelligence, it improves the process of locating tumors during radiotherapy treatment. These systems continuously analyze patient data, providing accurate details about the position and movement of the tumor. This ensures that therapy is delivered precisely, which is essential for both radiotherapy oncol and imaging purposes.
-
Artificial intelligence (AI) plays a crucial role in maintaining the integrity and consistency of imaging data used in radiation oncology. Automated machine tools are used for quality assurance processes, ensuring accurate acquisition, registration, and calibration of images for precise treatment planning.
-
With the integration of artificial intelligence and machine learning algorithms, radiation oncology clinicians can use AI-based adaptive radiotherapy to make real-time adjustments during treatment. This innovative approach combines imaging-guidance systems with neural networks to create personalized treatment plans that adapt to the evolving needs of individual patients.
Deep Learning for Auto segmentation in Radiotherapy Planning
Deep learning models, such as deep convolutional neural networks (CNNs), have revolutionized the field of radiation oncology by enabling the automatic segmentation of organs at risk and target volumes from medical imaging. This advanced technology significantly reduces manual effort, improves consistency, and accelerates the treatment planning process with the help of artificial intelligence.
One key advantage of using deep learning-based autosegmentation in imaging is that neural networks trained on large datasets achieve high accuracy levels comparable to human experts. These models leverage radiomic features and machine learning methods, such as deformable image registration, to accurately delineate target volumes, minimizing interobserver variability among different clinicians. The use of artificial intelligence, specifically DNN, enhances the precision and reliability of the autosegmentation process.
By replacing time-consuming manual segmentation with automatic delineation using deep learning techniques, such as neural networks (DNNs), radiotherapy planning becomes more efficient. This is particularly crucial in cases involving small-cell lung cancer patients or other complex anatomical structures where precise target volume delineation is essential for deformable image registration.
The implementation of deep learning algorithms in imaging enables deformable image registration, enhancing the accuracy of target volume delineation in radiation therapy. Through this technique, images from different time points or modalities can be aligned to account for anatomical changes during treatment.
Future Directions: AI’s Potential Impact on Radiotherapy
AI has the potential to revolutionize radiat therapy by improving treatment precision and patient outcomes in oncology. Here are some key areas where AI can make a significant impact on imaging and radiat therapy for oncology patients.
-
Tailoring Treatments: By leveraging AI algorithms, radiotherapy treatments can be tailored based on individual patient characteristics. This approach, known as precision medicine, takes into account factors such as tumor size, location, genetics, response to previous treatments, and imaging. The result is a more personalized and effective treatment plan that maximizes the chances of success for radiat patients.
-
Multi-Modal Data Fusion: AI algorithms can integrate data from various sources, including medical imaging scans, genomic profiles, and clinical records. By analyzing this comprehensive dataset, deep learning and machine learning algorithms can provide valuable insights into tumor behavior and predict treatment response more accurately. This multi-modal data fusion enables clinicians to make informed decisions regarding the most suitable course of action for each patient undergoing radiation therapy.
-
Decision Support Systems: AI-driven deep learning decision support systems have the potential to assist clinicians in making critical treatment decisions for patients. These systems analyze vast amounts of patient data and provide evidence-based recommendations for optimal treatment plans, leveraging the power of dnn. By accessing real-time information, clinicians can enhance their decision-making process and improve patient outcomes through advanced imaging techniques.
-
Collaboration between Researchers and Clinicians: The future of AI in radiotherapy relies on collaborative efforts between AI researchers and clinicians. Close collaboration ensures that AI tools for radiat imaging are developed with an in-depth understanding of clinical needs while incorporating deep learning and cutting-edge technological advancements. This synergy will shape the future landscape of radiotherapy by harnessing the full potential of artificial intelligence for patients.
Knowledge-Based Planning Solutions for Improved Treatment
Knowledge-based planning systems leverage artificial intelligence (AI) to generate treatment plans based on historical data. These systems analyze a vast amount of imaging, machine, and DNN treatment planning information and use predictive models to recommend optimized treatment plans for individual patients. By learning from past successful treatments, knowledge-based planning improves plan quality and reduces variations in radiation therapy.
These AI-powered solutions, using deep learning algorithms, provide valuable insights and recommendations for optimizing treatment delivery in radiotherapy. Clinicians can benefit from these tools by saving time and improving overall treatment efficiency for patients. Here are some key points about knowledge-based planning solutions in radiat therapy using dnn.
-
They utilize algorithms and machine learning methods to analyze large datasets of previous treatment plans for patients undergoing radiation therapy. This analysis includes segmentation techniques and can be found in research papers on Google Scholar.
-
The systems generate personalized treatment plans for radiation therapy patients that take into account tumor characteristics, anatomical structures, and clinical goals. The segmentation process helps to create accurate plans for oncol patients.
-
Knowledge-based planning solutions help clinicians make informed decisions by providing evidence-based recommendations for the best course of action for patients. These solutions utilize data from sources such as Google Scholar to ensure that the recommendations are backed by relevant research. Additionally, these solutions can also incorporate images to further enhance the decision-making process.
-
These deep learning-based approaches enhance clinical practice in oncology by streamlining the treatment planning process for radiation therapy patients, reducing the burden on clinicians. PubMed is a valuable resource for researching and accessing relevant information in this field.
-
Implementation of knowledge-based planning systems in the clinical workflow has shown promising results in terms of improved plan quality, reduced toxicity, and better patient outcomes. These systems utilize data from pubmed and google scholar to enhance radiation therapy for patients.
The Promising Future of AI in Radiotherapy
In conclusion, the field of artificial intelligence (AI) in radiation therapy is rapidly advancing, offering promising solutions for improved cancer treatment. Recent advances in AI have revolutionized various aspects of radiation therapy, including image-guided radiation therapy and autosegmentation in treatment planning. AI in radiation therapy is particularly beneficial for oncology patients undergoing treatment.
Deep learning has shown great potential in image-guided radiotherapy by enhancing the accuracy and precision of tumor targeting. By analyzing vast amounts of patient data, deep learning algorithms can identify patterns and optimize treatment plans to deliver more effective radiation doses while minimizing damage to healthy tissues.
Deep learning techniques have also been employed for autosegmentation in radiotherapy planning. These algorithms, utilizing radiat and ct, can automatically outline organs at risk and target volumes, saving time and improving consistency compared to manual segmentation methods. This has been supported by studies published on Pubmed, which demonstrate the efficacy of these techniques in oncol applications.
Looking ahead, the future of AI in radiotherapy holds immense promise for patients. Knowledge-based planning solutions powered by deep learning and AI can provide personalized treatment strategies for oncol patients. This approach has the potential to greatly improve treatment outcomes and reduce side effects. Read the full article to learn more.
To fully realize the benefits of deep learning in radiotherapy, collaboration between researchers, clinicians, and industry experts is crucial. By combining their expertise and resources, they can accelerate the development and implementation of AI technologies into clinical practice for the benefit of patients.
FAQs
Q: How does AI improve accuracy in image-guided radiotherapy?
AI uses patient data to make better cancer treatment plans. This helps target tumors and protect healthy tissues.
Q: What are the advantages of using deep learning for autosegmentation in radiotherapy planning?
Deep learning techniques automate organ outlining during radiation therapy treatment planning, saving time and ensuring consistency compared to manual segmentation methods. This is particularly beneficial for oncol patients who undergo CT scans as part of their treatment.
Q: How can knowledge-based planning solutions powered by AI benefit patients?
Knowledge-based planning solutions use segmentation and machine learning techniques to personalize radiation therapy treatment strategies for patients. These personalized strategies improve treatment outcomes and minimize side effects.
Q: What is the importance of collaboration in advancing AI in radiotherapy?
Researchers, doctors, and experts need to work together to make AI better for patients. They can use Google Scholar and PubMed to learn more.
Q: How can AI revolutionize cancer treatments?
By enhancing precision, efficiency, and personalization in radiotherapy, AI has the potential to greatly improve cancer treatments for oncol patients. This article explores how AI can benefit both oncol patients and phys in delivering better patient outcomes and potentially saving lives.