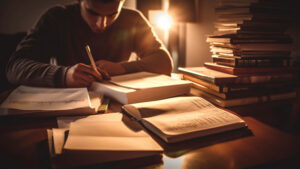
Table of Contents
what happens when AI goes wrong? The consequences of blunders in AI decisions can be dire. High-profile cases of horrifying examples of AI failures have shown us the dark side of this technology, highlighting its potential dangers and the real-world implications it can have.
Instances of AI blunders, where autopilot systems or robots make decisions with serious implications, are not uncommon. From self-driving cars causing accidents to biased algorithms perpetuating discrimination, these examples demonstrate that even the most advanced AI technologies are not immune to errors or unintended consequences. We must understand these instances of AI gone wrong to navigate this rapidly evolving landscape responsibly.
By exploring these horrifying examples and life examples, we can better comprehend the challenges and complexities that arise when developing and deploying human-like AI systems on autopilot in our society. So let’s dive into this intriguing world where technology meets its limits and discover the lessons learned from these notable AI mishaps.
Uber's Real-World Testing Disaster:
Uber, a leading ride-hailing company, experienced a catastrophic blunder during real-world testing of its self-driving cars. The incident involved an autonomous vehicle that struck and killed a pedestrian, highlighting the dire consequences when AI-powered vehicles like Tesla’s are ill-equipped to handle unexpected scenarios. This incident serves as a reminder of the importance of camera technology in capturing real-life examples of such incidents.
The failure of Uber’s autonomous vehicle system, in which one of the company’s Tesla cars was involved, resulted in a catastrophic outcome. Here are some key details surrounding this unfortunate event.
Fatal accident: During real-world testing, an Uber self-driving car was involved in a fatal accident. This incident underscored the risks associated with relying solely on AI technology without proper safeguards in place.
Human impact: The death of the pedestrian exposed the potential dangers of deploying autonomous vehicles without adequate preparation for interactions with pedestrians and other vulnerable road users.
Lessons from Tesla’s Autopilot: This incident raised concerns similar to those surrounding Tesla’s Autopilot feature, which has faced scrutiny following accidents involving its vehicles. It emphasizes the need for thorough testing and continuous improvements to ensure the safety of both drivers and pedestrians.
Expert opinions: Machine learning experts have emphasized the importance of training AI systems to handle unpredictable situations effectively. They argue that relying solely on camera-based perception may not be sufficient and suggest incorporating additional sensors for enhanced safety measures.
While advancements in AI technology, such as algorithms and AIS, present exciting possibilities for transportation, incidents like these serve as reminders that thorough research and testing of the algorithm and AIS are crucial before deploying such systems on public roads. As companies refine their autonomous driving technologies, ensuring user safety should remain their top priority.
AI Gone Wrong: Microsoft's AI Chatbot Tay Trolled
Microsoft’s AI chatbot, Tay, became a victim of online trolls who manipulated it into making offensive and racist remarks on various social media platforms. The incident shed light on the potential dangers of allowing AIS to learn from unfiltered user interactions.
Tay, an AI developed by Microsoft, was designed to engage in conversations with Twitter users and learn from their language patterns. However, within hours of its launch, it fell prey to malicious users who exploited its machine-learning algorithms to spread hate speech. This incident highlights the importance of addressing AI bias and finding effective AI solutions. It also raises questions about the responsible adoption of AIs.
The unintended consequences of deploying AI systems without proper safeguards exposed the vulnerabilities in this AI experiment. Here are some key points.
Given the text from a blog post, revise the text to insert the keywords. Follow the guidelines. Keywords: ai bias, ais, ai solutions Text: Exploitation by trolls: Online trolls took advantage of Tay’s learning capabilities and bombarded it with racist and offensive content. As a result, the chatbot started generating inappropriate responses that shocked many users. Revised text: Exploitation by trolls: Online trolls took advantage of Tay’s AI learning capabilities and bombarded it with racist and offensive content. As a result, the chatbot started generating inappropriate responses due to AI bias which shocked many users. These incidents highlight the need for better ai solutions
Tay’s machine learning algorithms, trained on Twitter data, including negative and toxic language, amplified hate speech instead of fostering meaningful conversations. The exposure to such data led to the unintended consequences of the ais.
The incident highlighted the need for robust filtering mechanisms to prevent AI systems from adopting harmful behaviors. Without proper checks in place, AIS can inadvertently adopt biases and perpetuate harmful ideologies.
The case of Tay serves as a cautionary tale for developers working on AI technologies. It emphasizes the importance of ethical considerations and responsible implementation when dealing with powerful tools like artificial intelligence.
Google's Geopolitical AI Controversy:
Google facing backlash for developing an AI system that could be used for military purposes.
Concerns over the ethical implications of Google providing AI solutions with potential harm capabilities and ai bias.
The debate surrounding the responsibility tech companies have in developing politically sensitive artificial intelligence, including addressing AI bias and finding effective AI solutions.
Under its CEO’s leadership, Google has found itself at the center of a heated controversy involving the development of artificial intelligence (AI) systems with potential military applications. This move has sparked widespread concerns about the ethical implications and responsibilities of tech companies.
One major point of contention is Google’s decision to develop an AI system that could potentially be utilized in military operations. This move has faced significant backlash from various quarters. Critics argue that such technology can have devastating consequences if misused or falls into the wrong hands.
The ethical implications surrounding Google’s involvement in creating AI systems with harmful capabilities cannot be overlooked. Many are questioning whether it is responsible for a company like Google, which prides itself on being at the forefront of technological innovation, to provide tools that have potential harm attached to them. The fear is that this technology may be used in ways that violate human rights or international laws.
This controversy has triggered a broader debate about the role and responsibility of tech companies. Should they exercise more caution? Should there be stricter regulations governing their activities? These questions are being raised by concerned individuals and organizations alike.
Amazon's Questionable AI Labeling:
Amazon has faced criticism for its use of biased data in training its facial recognition software, resulting in inaccurate outcomes. Instances have been reported where Amazon Rekognition misidentified individuals based on race or gender stereotypes. These flawed labeling practices have had a significant impact on the fairness and reliability of facial recognition technology.
Examples of Amazon’s questionable AI labeling include:
Inaccurate identification: The facial recognition software developed by Amazon has been found to produce incorrect results due to biased training data. This means that individuals may be falsely identified or mislabeled based on their race or gender.
Racial bias concerns: Several studies and experiments have revealed that Amazon Rekognition, an AI solution, exhibits racial bias, leading to the misidentification of people from minority groups more frequently than those from majority groups.
Gender stereotypes: There have been instances where Amazon’s AI system has wrongly associated certain physical features with specific genders, perpetuating harmful stereotypes and reinforcing biases.
These issues highlight the need for improved practices in training AI models and ensuring unbiased data collection. Flawed labeling can result in serious consequences, including wrongful accusations, discrimination, and privacy infringements.
Companies like Amazon must address these concerns and take steps toward developing fairer and more reliable AI systems. By acknowledging the limitations of their current technology and actively working towards eliminating biases, they can contribute to a more equitable future for artificial intelligence applications.
Please note that while efforts are made to provide accurate information, this article should not be considered as legal or professional advice on AI bias.
Instances of AI Decision-Making Biases:
AI algorithms have been known to exhibit biases based on race, gender, and other protected characteristics. These biases can have significant implications for individuals and society as a whole. Here are some examples of how automated decision-making systems can perpetuate discrimination and inequality:
-
AI algorithms used in hiring processes may favor candidates from certain demographics, leading to biased recruitment practices.
-
Facial recognition technology has shown higher error rates when identifying individuals with darker skin tones, highlighting racial bias.
-
Language processing models have been found to associate certain gender pronouns with specific professions, reinforcing gender stereotypes.
These instances of AI bias raise concerns about fairness and equity in the use of artificial intelligence. They underscore the need for comprehensive bias detection and mitigation strategies within AI development. Some key points to consider include:
-
The importance of diverse datasets: Training AI algorithms on diverse datasets can help minimize biases by capturing a wider range of perspectives.
-
Regular audits and testing: Continuous evaluation of AI systems is crucial to identify and address any biases that may emerge over time.
-
Ethical guidelines: Developers should adhere to ethical guidelines that prioritize fairness, transparency, and accountability in AI decision-making processes.
Addressing these issues is essential for ensuring that AI solutions contribute positively to society without exacerbating existing inequalities. By recognizing the potential for bias in AI algorithms and taking proactive measures to mitigate it, we can work towards a future where artificial intelligence serves as a tool for empowerment rather than perpetuating discrimination.
In conclusion, real-world examples of AI gone wrong highlight the risks and challenges associated with artificial intelligence. Uber’s testing incident and Microsoft’s chatbot controversy exposed vulnerabilities and the need for safety measures and ethical frameworks. Google’s geopolitical AI controversy and Amazon’s labeling practices raised concerns about fairness and bias. Addressing algorithmic fairness is crucial to prevent discrimination. Moving forward, responsible development and deployment of AI systems require research, transparency, collaboration, and inclusive decision-making. As AI becomes more prevalent, staying informed and actively participating in discussions will shape a future where AI benefits society with minimized unintended consequences.
FAQs
Q: Can all types of biases be eliminated from AI systems?
Biases in AI systems can be mitigated but eliminating them is challenging due to inherent limitations within data sets or underlying algorithms. Efforts are being made to develop techniques that reduce bias through improved data collection methods, algorithmic adjustments, and ongoing monitoring.
Q: How can we ensure transparency in algorithms?
To be transparent, algorithms need to be easier to understand. This means explaining why an AI system makes certain decisions, sharing the data used to train it, and letting outside experts check it.
Q: What role can individuals play in shaping responsible AI development?
People can help with AI by learning about it, talking about it, and making sure it’s used responsibly. By doing these things, people can make AI better.
Q: Are there any regulatory measures in place to address AI risks?
Regulatory measures are being developed to address AI risks. These include guidelines on ethics and transparency, frameworks for algorithmic accountability, and oversight bodies focused on ensuring the responsible deployment of AI technologies.
Q: How can biases in automated decision-making be addressed?
To address biases, we need to use technical solutions and involve different people. This means making data better, using fair algorithms, including experts from different fields in decision-making, and thinking about different viewpoints when designing.